Retail media has become a red-hot space for advertisers for a good reason. Digital retail platforms attract large audiences of target customers and personalize their shopping experience. They have tons of customer data at their fingertips to reach shoppers at the point of sale and influence purchase decisions.
Retail media platforms use this data to inform advertising campaigns with detailed insights. They analyze collected information on shopping behavior, consumer preferences, and purchase patterns. The incremental value of these efforts is that advertisers can improve the ROI of their media ad spend and outrank their peers. Retailers, in turn, can improve monetization opportunities, predict future results, and measure their performance.
However, while many retailers praise the role of Big Data and analytics, most need help to incorporate it in decision-making. That’s because analyzing the flood of information, which can overwhelm many businesses, is necessary. Retailers need a data foundation with robust retail media analytics capabilities to succeed.
How does data analysis aid in improving the bottom line of retailers? What is the role of advanced technologies in this process? Which aspects can limit the potential of data analytics? We’ll explore these and other considerations in the sections below so that you gain a better grasp of this vibrant topic for your retail media company.
What Retail Media Data Analytics
Helps With
Understand customers through unified data
Retailers have the privilege of using valuable first-party data to tailor their promotional and advertising activities to the needs of individual customers. They collect accurate information and benefit from the exclusive nature of direct relationships with their audiences. With third-party cookies undergoing gradual transformation due to privacy-focused data collection approaches, first-party data creates a firm ground for understanding a modern consumer.
Metrics | What’s included | Impact |
---|---|---|
Demographics | Age, gender, location, income | Segment audiences by specific groups |
Purchase history | Dates, product types, quantities from past purchases | Understand individual preferences |
On-site behavior | Visited pages, CTRs, time spent browsing for products | Adjust content |
Shopping frequency | Regularity of online and offline shopping | Identify loyal customers and their attributes |
Channels | Data on interactions across all channels | Understand customer journey |
Engagement level | Open rates, dwell time, clicks, QR code scans, etc. | Assess interest from users |
Abandonment rates | Products added to cart without purchasing | Identify roadblocks in the shopping flow |
Customer feedback | Reviews and ratings | Inform service improvements |
The above metrics are critical for identifying patterns in user behavior and making conclusions about their standard and distinctive features. However, this data should flow in a central location so that each department can access and analyze it in one place. Otherwise, it will remain siloed in different systems and platforms and won’t become a single source of truth.
The solution to this problem is adding a CDP (Customer Data Platform) to your retail media tech stack. As one of the best ways of unifying first-party data, a CDP allows retailers to dive into a 360-degree view of their customers. It helps reveal how consumer groups browse, shop, and interact with ads and other content elements.
A thorough analysis of the insights generated by a CDP helps target specific audiences with resonating retail media ads. For example, suppose a CPG retailer notices that a particular group of repetitive customers buys organic products. In that case, they can use a CDP to segment these customers and advertise brands that provide sustainable product lines.
Make decisions based on data
Data analysis provides logical backing for almost all aspects of retail media operations. Instead of relying on assumptions and intuition, it considers fact-based insights. The insights help identify helpful information, inform business choices, and support decision-making.
The process behind is fine-tuned to transform raw data into digestible formats. For these purposes, data undergoes several critical stages: collection, cleansing, transformation, and modeling.
Step | Overview | Example in retail media |
---|---|---|
Collection | Collect raw data from available sources | Log ad interactions, impressions, session duration, etc. |
Cleansing | Address errors, duplicates, and format inconsistencies | Ensure accurate and consistent tracking of ad impressions |
Transformation | Convert data into usable formats: visualizations and benchmarking | Create applicable metrics: CTRs, conversion rates, etc. |
Modeling | Apply models to identify patterns and predict future outcomes | Predict LTV and adjust bid strategies |
Deriving insights | Interpret key findings | Recommendations on adapting campaign settings |
Similarly, data analysis can guide the following aspects:
- Ad inventory management. Data-driven decisions help improve the performance of ad inventory. For example, if a particular ad format generates more clicks than the other, retailers can serve it more frequently and charge advertisers a premium rate.
- Product recommendations. Data analysis can reveal what’s hidden when product recommendations fail to resonate with consumers. It helps suggest relevant retail media offerings based on previous shopping experiences and behavioral patterns.
- Promotions. Armed with accurate data on peak traffic, retailers can launch exclusive ad campaigns with premium-priced media offerings. A surge in customer interaction during specific time slots helps capitalize on improved conversions and heftier price tags for ad inventory.
Our retail media analytics services will help you leverage clean and structured data to back your decisions with actionable insights.
Personalize marketing campaigns
Data is a powerful engine for creating hyper-personalized marketing campaigns across all the stages of the shopping funnel. Retailers can leverage a single view of their customer's interests and preferences and feed this information into AI-driven marketing platforms. They, in turn, can process vast amounts of data and make real-time decisions when communicating with each customer.
Unlike traditional segmentation, which groups customers by common traits, hyper-personalization helps reach customers individually. AI formulates insights from live behaviors, psychographics, and engagement models. This approach lets brands serve unique experiences through:
- personalized product recommendations,
- special discounts,
- dynamic pricing, etc.
Stage of the funnel | What users see | Role of data analytics |
---|---|---|
Awareness | Relevant ads on the web | Audience segmentation |
Consideration | Personalized promotions on a landing page | Insights into past interactions |
Intent | Tailored recommendations | Analysis of past purchases |
Evaluation | Dynamic prices and products | Analysis of preferences and cost consciousness |
Checkout | Real-time support | Personalized assistance via chatbots |
Post-purchase | Follow-up retail media ads | Data integration from all touchpoints |
Loyalty | Custom promotions | Purchase data is used to encourage repeat buying |
Balance retail media ad spend
When retailers allocate advertising budgets without understanding which channels, strategies, inventory types, and creatives deliver the best and worst results, money is wasted. This situation happens because companies don't measure the impact of their advertising on revenue and sales.
That's where data analytics delivers outstanding results in ad spend optimization. Unlike traditional advertising channels, retail media platforms connect the dots between ad impressions and actual purchases. Moreover, they excel at identifying attribution models. As a result, brands can track the campaigns that are most likely to convert.
Data analytics also contributes to ad spend optimization in the following ways:
- It helps discover high-performing campaigns
A lot of moving parts contribute to labeling a campaign as high-performing. Retailers can use testing methods to detect the campaigns that bring tangible results. For example, A/B testing helps serve different versions of the same creative to live audiences and detect their top-converting elements.
In retail media measurement, monitoring pertinent metrics is also crucial. Tracking various benchmarks to fully understand campaign performance is necessary as it considers the current situation from multiple angles. Real-time data analysis helps significantly in this context by enabling continuous and immediate adjustments instead of waiting for post-campaign reports.
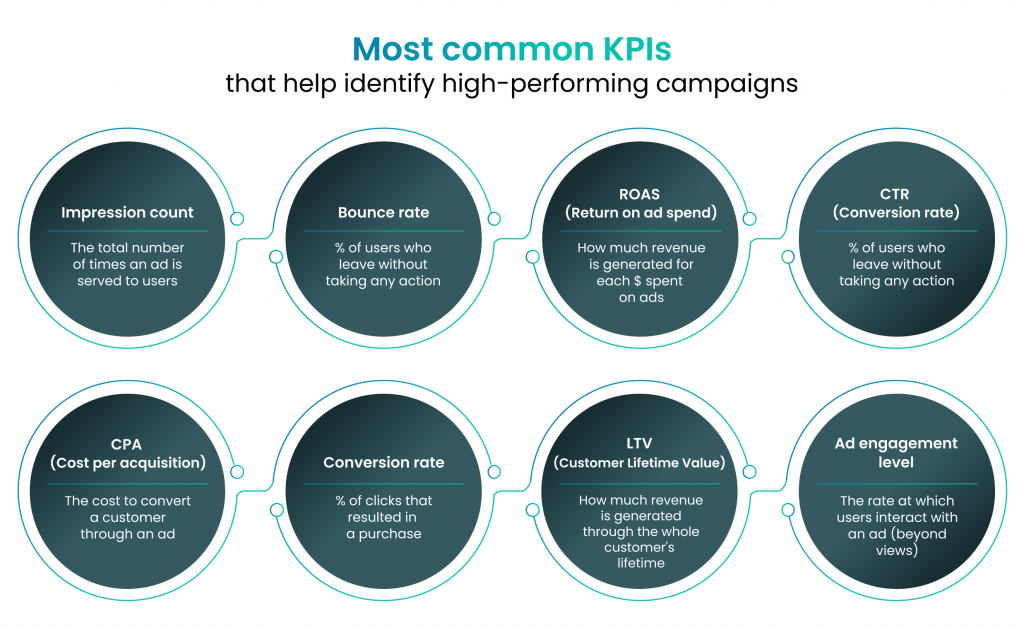
Continuous analysis of these metrics leads to more efficient budget allocation. Budget wastage becomes less frequent as investments flow to top-performing campaigns.
- It helps improve low-performing campaigns
By recognizing trends like low click-through rates (CTR) or high bounce rates, retailers can pinpoint areas that require attention. They can take measures to refine ad creative, adjust targeting parameters, or repurpose the budget towards more efficient campaigns.
Our senior software engineers are ready to design a data analytics solution that helps increase the ROI of retail media campaigns. Eliminate wasted ad spend and target your audiences with extra precision.
How Advanced Technologies Transform Data Analysis in Retail Media
The wealth of retail media data has urged businesses to adopt new ways of activating and processing it. If previously demand for innovative tools outstripped supply, the tables have turned. We can currently observe widespread adoption of AI, ML, predictive modeling, and automation. These innovations help yield incomparable results in data analysis for retail media and beyond.
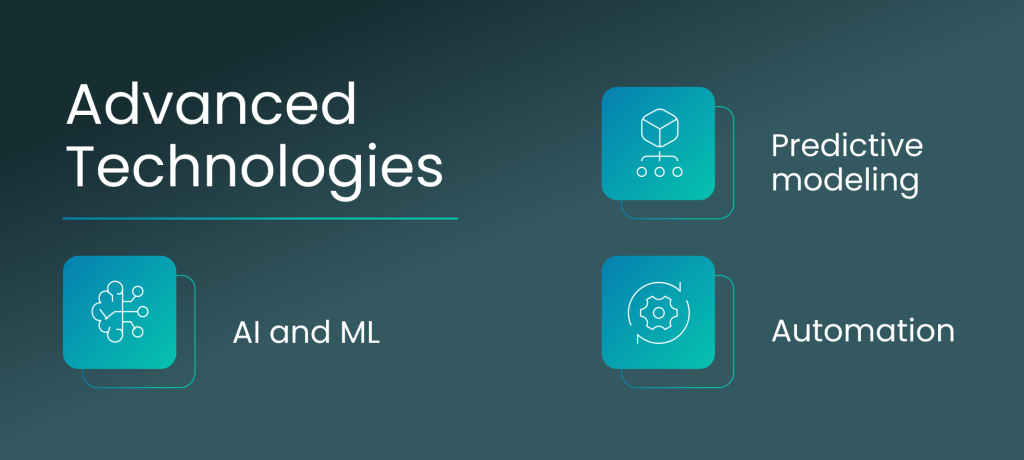
AI and ML
AI-driven retail media analytics for business helps attain insights that wouldn’t be possible with other methods. Artificial intelligence can collect and activate data at scale, which is beyond a human’s manual capability at the current moment. Here are the ways retailers use AI and ML to improve data analysis:
- Process extensive amounts of data and generate insights in the shortest time frames;
- Detect patterns in complex data sets and unstructured data;
- Improve the democratization of data;
- Enhance the quality and accuracy of data integration, etc.
Predictive modeling
Predictive modeling relies on historical data, statistical algorithms, and ML to forecast possible outcomes. Retailers can anticipate future customer behavior, sales trends, and demand for specific promotions or products. Here’s how they use predictive models:
- Learn from all available data to predict responses to particular campaigns;
- Identify which customer segments are likely to churn and take proactive measures to re-engage them;
- Analyze the competitive landscape and consumer demand to implement dynamic pricing models;
- Optimize ad spending by identifying campaigns that generate the highest ROI, etc.
Automation
Automation unlocks powerful capabilities for retailers willing to streamline their data analytics workflows. It aids in processing large data sets, delivering real-time insights, performing repetitive tasks, and reducing manual work. Here’s how retailers can use automation to accelerate data analysis:
- Create comprehensive reports on campaign performance;
- Analyze purchase and consumer behavior patterns;
- Put routine tasks on auto-pilot;
- Combine with AI to automate data analysis;
- Support predictive modeling processes, etc.
Examples of How Retailers Use Data Analytics to Inform Their Strategies
Retailers embrace the potential of data analytics to make informed decisions in different aspects of their operations. Data-driven strategies help them meet consumer expectations, launch hyper-personalized advertising campaigns, and improve engagement rates.
Let’s have a look at how the top names in the retail industry leverage data analytics:
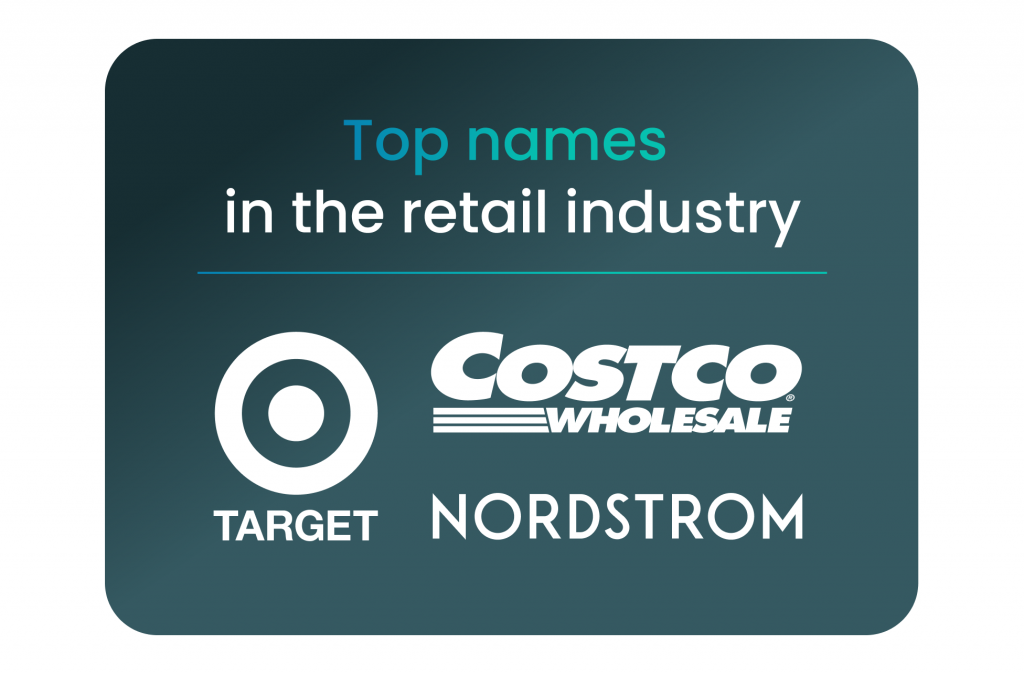
- Target analyzes its clients' purchases and browsing history using data analytics tools. Next, it leverages the insights obtained to inform the product recommendation engine. As a result, the retailer launches and scales personalized product suggestions that resonate with individual consumers.
- Costco capitalizes on first-party data by detecting patterns in user behavior. The company unifies all data from various touchpoints to refine pricing strategies, tailor product recommendations, and manage inventory levels.
- Nordstrom adopted a data-driven strategy to improve customer engagement and loyalty across the entire ecosystem of multinational stores. This renowned retailer uses data analytics to collect personal information related to style preferences and shopping patterns. Next, it customizes user experience to meet individual needs.
Challenges in Retail Media Analytics
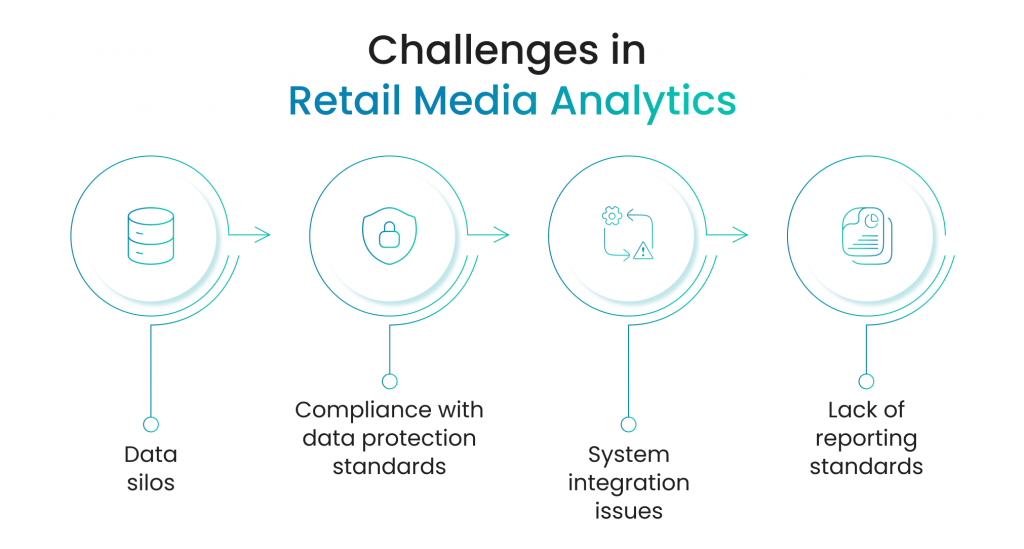
- Data silos
Retailers typically deal with data scattered across disconnected systems and platforms. Behavioral, transactional, and demographic data can reside in isolated folders. Sales data, customer feedback, and inventory levels can also be locked in standalone departments.
The variety of sources makes it difficult to have a 360-degree view of valuable data. Retailers can address this issue by integrating them into one interconnected system. They can implement a centralized data collection infrastructure and employ advanced tools for automated data integration.
- Compliance with data protection standards
Businesses need help to adhere to laws and regulations protecting privacy rights. Analytically savvy retailers often have multiple departments that collect sensitive data differently. Many companies also operate in regions and countries with contrasting data protection policies.
Compliance with evolving regulations requires careful thought. Retailers must incorporate rigorous standards, establish a secure data storage system, and train employees on the best data management and utilization practices.
- System integration issues
Data extraction and interpretation become complicated when analytics platforms operate in isolation from other elements of the tech ecosystem. For example, eConsultancy found that only 23% of retailers share live campaign data with their partners. Data exchange roadblocks lead to delayed decision-making.
Integration with the retail media tech stack is one of the top priorities for retailers. Seamless data sharing with marketing platforms, AdTech solutions, and retail media APIs will enable uninterrupted access to the most valuable data sets.
- Lack of reporting standards
As a Nielsen and Coresight research paper stated, more than half of respondents face significant reporting challenges due to a lack of established norms. This situation leads to misalignment between retailers and advertisers. With uniform metrics, accurate measurement of ad campaign results becomes more effortless.
Retailers can collaborate with industry peers to develop comprehensive reporting standards. They can develop intuitive dashboards that provide advertisers instant access to campaign performance data.
Conclusion: What the Future Holds for Data Analytics in Retail Media
The future of data analytics in retail media is ripe with powerful capabilities. Instead of relying on guesswork, retailers can use data as an ally to micro-target and hyper-personalize advertising messages in offline and online settings. They can outsmart the growing competition with game-changing solutions based on factual information, real-time insights, and exclusive knowledge of their target audiences.
Adapting to the growing adoption of data analytics requires embracing cutting-edge technologies. Retail media evolves at a similar pace as AI, ML, and predictive analytics, which presents new opportunities for retailers, brands, and advertisers. Those who quickly incorporate emerging data analytics capabilities into retail media networks and other platforms will likely succeed in the coming years.
As a company with 14+ years of experience in retail media, digital advertising, and Big Data analytics, Geomotiv is the right development partner for your project. We are up to the challenge if you want to enhance your retail media solution with advanced data analytics capabilities.
With our help, you can:
- develop personalized user experiences to engage and convert your target customers;
- unify disparate data sources to have a single view of all information related to your audiences, ad campaigns, and partners;
- optimize yield and implement ROI-generating monetization approaches;
- launch omnichannel campaigns and analyze their performance in intuitive dashboards;
- avoid compliance issues and build trusting relationships with all ecosystem partners.
Contact us to learn more about our offerings and start reaping the benefits of data-driven retail media solutions.
FAQ
We Help Unlock Answers to Your Questions
Retail media analytics transforms raw data on customers, sales, and ad campaigns into insights that inform decisions.